Global Vegetation Index (NDVI), annual variation
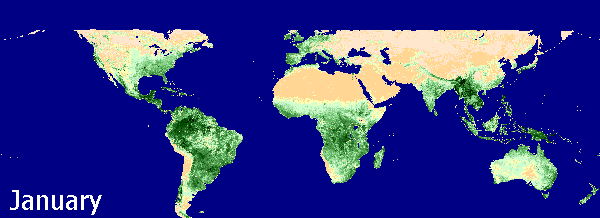
Sensing Systems for Precision Farming
Our goal in sensor development research is to develop and evaluate new ground-based sensing tools to
increase data quality and validate remote sensing systems for precision farming. We have investigated
the feasibility of using sensor (data) fusion technologies to develop dependable crop system monitoring
tools (decision support systems).
The potential of precision agriculture is limited by the lack of appropriate measurement and analysis techniques for agronomically important factors*. While the concept of precision farming is sound, our understanding of the physical and biological aspects of the cropping system is incomplete due to limitations in the current sensing and data processing technologies. Obtaining and analyzing data are the bottlenecks in the traditional system. The cost of obtaining information through traditional means (e.g., sampling for soil fertility or pest presence) is expensive and time consuming, and data collection is usually conducted in a sparse manner. The limitation in data quality/availability has become a major obstacle in the demonstration and adoption of the precision technologies. The development of sensors lags behind other enabling technologies. High accuracy sensing tools must be developed and validated to support both research and production.
Ground based sensing system
To use machine vision systems in outdoor fields the special difficulties of an unstructured work environment must be addressed. Light source temperature is one of the key issues that must be carefully studied. Variability in light source temperature causes the colors of objects in the image to change, making image segmentation very difficult. Our major contribution in this area is the development of a series of outdoor field image segmentation algorithms. The variability associated with outdoor lighting condition changes necessitates an environmentally adaptive segmentation algorithm (EASA) which can adapt to these lighting condition changes by using different look-up-tables (LUT) and making camera adjustments. The segmentation performance of the EASA specification stage was improved through the development of the reduced dimension clustering (RDC) algorithm and subsequent classifiers making this procedure unsupervised where it was partially supervised in earlier research. The RDC incorporated knowledge about the structure of the image data based on reflection models and observations to accomplish these improvements. Also coming out of these improvements was a method with the capability of estimating global features of the image data. These features show promise for use in the characterization of sensors. In addition, LUT visualization techniques were developed which are useful in showing how particular classifiers divide the color space.
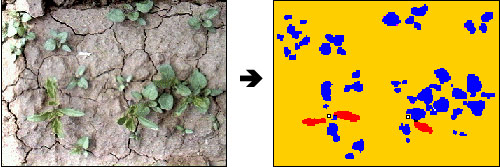
Hybrid positioning sensors
In this project, we were trying to use sensor fusion technology to increase field location sensor accuracy. We introduced a GPS-encoder hybrid positioning system. This hybrid system was composed of a differential GPS receiver and two optical encoders on the vehicle shafts. Integrating the GPS signal with the optical encoders output by curve matching can significantly improve the positioning resolution in both global range and single-point. Since the matched curve is based on the directional information from GPS and the curve shape from the optical encoder, the resulting resolution is much higher. The system retains the advantages of GPS and optical encoders, such as low cost, high-speed response and high resolution. To evaluate and validate our new system, we have developed a unique machine vision based true path generator. The experiment results revealed that with the new hybrid system, the positioning accuracy of a ground truth machine has been significantly improved. The sensing system can meet the real-time spatial high resolution positioning demands in field operations with the high-speed dynamic response.
Agricultural remote sensing
Our approach is to introduce new sensing technologies to evaluate and validate remote sensing for field variability
identifications. With support form the NASA Commercial Remote Sensing Program (CRSP), we developed a general-purpose
ground-based sensing system to collect high-density ground truth data for the site-specific and time-specific
validation of remote sensing as a field characteristic mapping tool for major crop production. We have built a
low-altitude high-resolution aerial imaging system. Using an airborne high-resolution digital camera and a real-time
image processing and pattern recognition system, we have investigated some fundamental problems related to
new high-resolution remote sensing techniques. While satellite remote sensing systems can only fly over a site
at a certain time and obtain a spatial resolution of several meters per pixel, our system is near real-time and
the ground truth machine can obtain images with much higher spatial resolution. This near-real-time system enables
us to relate image patterns to ground truth more closely. More details about the targets have been found through image
processing (Pillai and Tian, 1999). Our aerial multi-spectral data was successfully used to identify crop nitrogen
stressed field areas and predict the corn yield accurately with an R2 value of 0.96. Unlike other methods that only
predict for whole fields, our remote sensing data can be used to generate detailed in-field yield variability maps.
One important outcome from this research is that we discovered the spatial resolution of aerial imaging system
should be a function of ground truthing accuracy. With our high accuracy ground truthing machine, the practical
spatial resolution of the remotely sensed data should be much better than 3 meters per pixel.
Because of the potential of agricultural remote sensing, NASA-USDA has announced the "AG 20/20," a five-year program
targeted for $17 million in funding starting in FY2001, to promote remote-sensing-based tools for improving profit margins
for commodity producers. As part of the NASA-CRSP Strategic Plan our remote sensing project has been selected as
a "preliminary Project." The Illinois Council on Food and Agricultural Research, Inc. (C-FAR) has awarded one
million dollars of its new Sentinel Program to support the agricultural remote sensing program in the College of ACES.
A new remote sensing laboratory is going to be built to implement the state-of-the-art high-performance computing system
to develop special image analysis tools for multi- and hyper-spectral field image processing and archiving of image pattern data.
My next-step research effort will be concentrated on quantitatively evaluating and validating remote sensing systems for different precision farming applications. We will use ground-based sensor data to determine the possible information loss of lower-resolution remotely sensed data, and simulate possible new remote sensing system design with high spatial (temporal or spectral) resolution ground-based images.